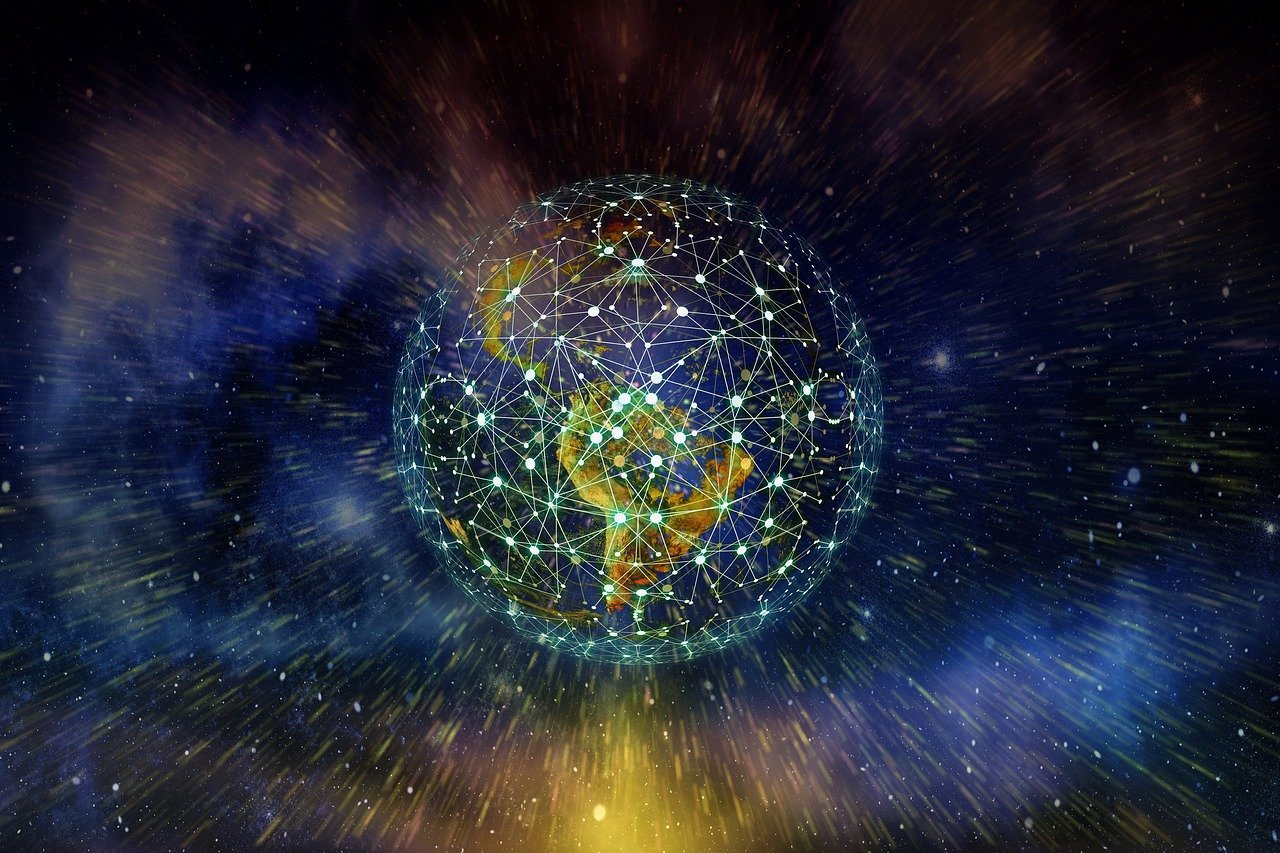
Semantic Technologies for Steel
Task 20 Team
-
Prof. Cinzia Giannetti
Swansea University
-
Prof. Arnold Beckmann
Swansea University
-
Dr Rahulan Radhakrishnan
Swansea University
Introduction
Data is everywhere, but not always very usable. This is particularly true in the steel industry, which collects large volumes of data daily. However, the industry is behind in exploitation of data assets. Steel plants are large scale and complex; many operate utilising legacy equipment alongside newer machineries, hence are often challenged by poor data quality. They rely heavily on expert operators and metallurgist to achieve optimal process operations. This makes data mining difficult and poses a huge challenge for applying advanced data analytics and other AI technologies. Current data and AI approaches are often used to optimise single assets or processes, resulting in challenges for their integration and subsequently sub-optimal operations, which in turn leads to loss of profitability and detrimental effects on the environment.
A promising way to address this is to employ semantic technologies. This aims to give meaning to all kinds of digital information, to make that information understandable for machines to enable advanced information processing and reasoning. Semantic technologies have been very successful in digitising human knowledge in expert systems, and have successfully been used to reason about knowledge, data and processes in industrial domains.
Task 20 will address 5 key areas of research:
Ontology refinement, data integration and hybrid predictive analytics. A fundamental task is working with a partner to adapt the core reference ontology to their processes. This will refine the ontology and demonstrate data integration.
Ontology refinement for through process modelling. Research will also consider through process modelling from cast steel slab to finished coil. Based on expert knowledge, the core reference ontology will be refined. The main aim will be to apply predictive analytics to key product properties.
Semantic support for AI for science. Machine Learning will be investigated to increase efficiency of scientific modelling. This will be enhanced semantically by developing frameworks that allow for the integrating of additional knowledge like expert knowledge.
Digitalisation of sustainability assessments. The research will aim to provide a semantic conceptualisation of LCA / TEA relevant for the steel industry. This will build on recent work which provides a core reference ontology for LCA2, and will refine that approach in the context of Task 18 to provide a semantic view on the Sustainability Toolbox.
Material passport conceptualisation. Work will aim to conceptualise core elements of a material passport, by developing accompanying semantic concepts to improve management and enable exchange of information
The research will enable evaluation of novel hybrid approaches to enhance current modelling and data analytics capabilities, allowing exploitation of hidden potential of existing data assets to drive productivity improvements and sustainability. Task 20 will provide a set of ontologies, data integration methods and guidelines that will enable integrate humans’ decision making and the vast tacit knowledge within existing modelling and analytics frameworks. The project will contribute to enhance our understanding of the application of hybrid methods in manufacturing, which is a relatively unexplored field. This will ultimately leading to improved decision-making capabilities and optimal operations.
Progress to Date
We are currently exploring various aspects of semantic technologies for integration into the data and knowledge management process of the steel manufacturing industry. Below are the key developments to data:
Ontology Development:
Refined the existing steel ontology within SUSTAIN: CROS – Common Reference Ontology for Steelmaking.
Developed the Hot Rolling Mill Process Ontology by detailing sub-processes, machinery, data properties, energy, and emission flows within the Hot Rolling Process (Figure 1).
Identified key concepts of Life Cycle Assessment such as Process, Flow, and attempted to incorporate them within the Hot Rolling Mill Ontology (Figure 2).
Explored data from the Hot Roll Milling process and categorized them into three types based on accessibility, availability, and usability within the industry.
Data Management:
Demonstrated efficient data integration within the steel industry using knowledge graphs.
Integrated large language models into our semantic research framework.
Conducted preliminary studies on utilizing large language models for data integration, querying, and analysis within the industry.
Proposed research to develop an enhanced data integration and querying framework for the steel manufacturing industry using the power of large language models.
Digital Material Passport for Steel:
Conducted a literature review.
Developed an initial conceptual framework for a Digital Material Passport for Steel using Ontologies, knowledge graphs, and large language models (Figure 1).
Figure 1: Digital Material Passport Conceptualisation
Figure 2: Steel LCA ontology (initial development)
Next Steps
In the next stages, we will expand the hot rolling mill ontology to accommodate the variations in processes among different steel-making companies. This expansion will be validated with input from industrial experts. Additionally, we will conduct further work on a real-world case study with our industrial partners to validate our semantic framework. This framework incorporates large language models for data integration, querying, and analysis, ensuring its effectiveness and relevance in industrial settings. Furthermore, we will continue our study on Digital Material Passports, aiming to strengthen the concept and enhance its applicability within the steel industry.